Deep Relief: Painterly in the Age of Brute-force Computing
Deep Relief is an architecture project that seeks to reassess the concept of the painterly traits integrating brute-force computation and robotic fabrication in the design of an architectural wall relief. Using a hot-wire cutter attached to a 6-axis robotic arm, a single line is positioned and swept through space to carve ruled-surface geometry in foam. The resultant hyperbolic surfaces curate gradients of light and shadow effect. When viewing a photograph or rendered two-dimensional flat image of the carved figure, the embedded three-dimensional traits of construction can be mined: the ruled geometry of the surface, the sweeping directionality, and the choreography of robotic arm’s motion.
A VGG-19 deep learning neural network and cloud-based computing are used to produce non-authorial compositional images of these understandable traits. Even though the convolutional neural network redistributes and hybridizes the hierarchical features of the ruled surface geometries in unimagined ways, the information and localized robotic motion can be reconstructed to fabricate a novel three-dimensional relief. The resultant Deep Relief project embodies a whole new and non-intuitive post-human hybridization of part to whole, expressing the current architectural paradigm: painterly in the age of brute-force computing.
[1] Wölfflin, Heinrich. Renaissance and Baroque. Fontana Library. [Renaissance und Barock.]. Vol. [873L]. London: Collins, 1964, pp. 77.
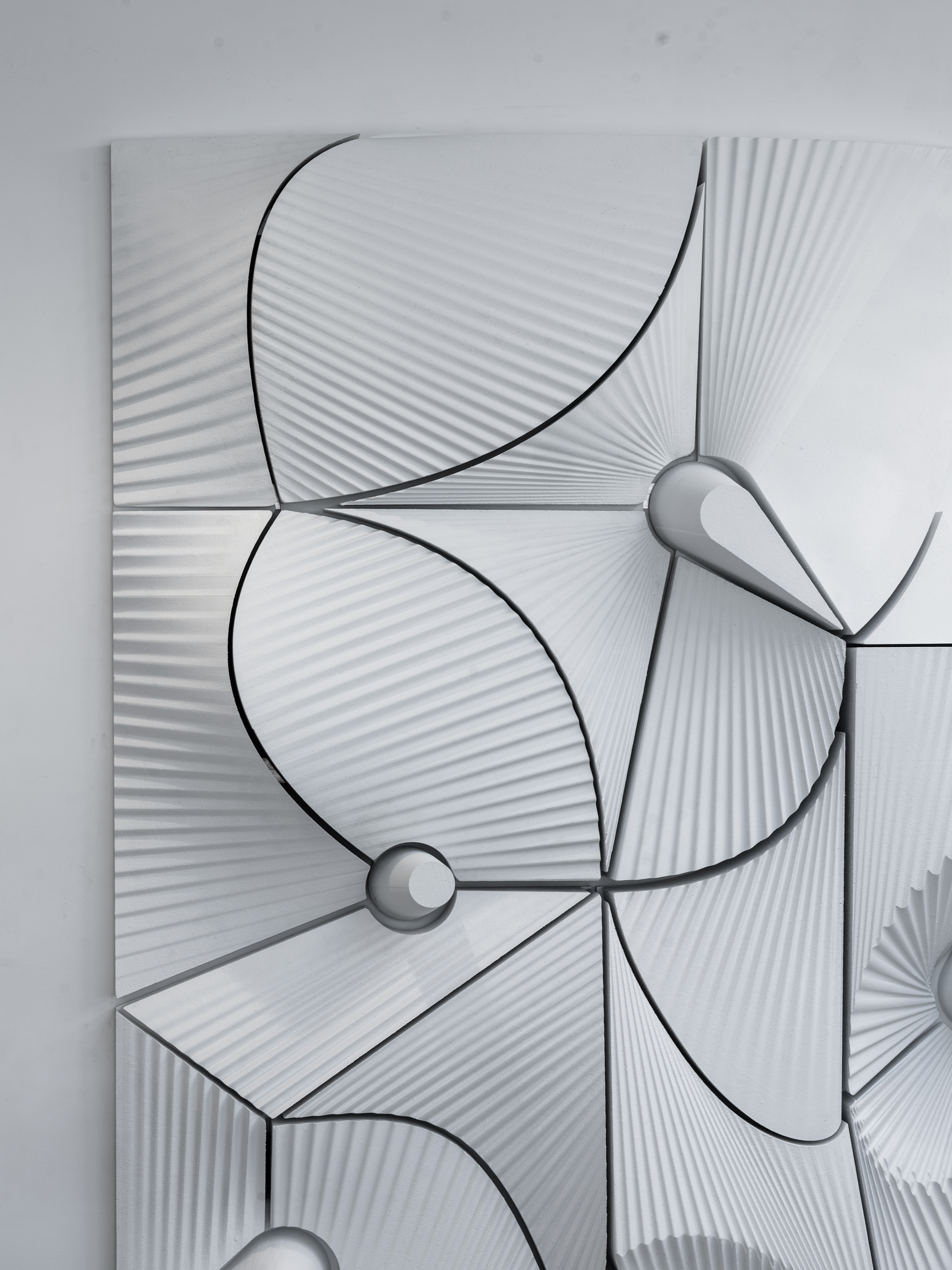
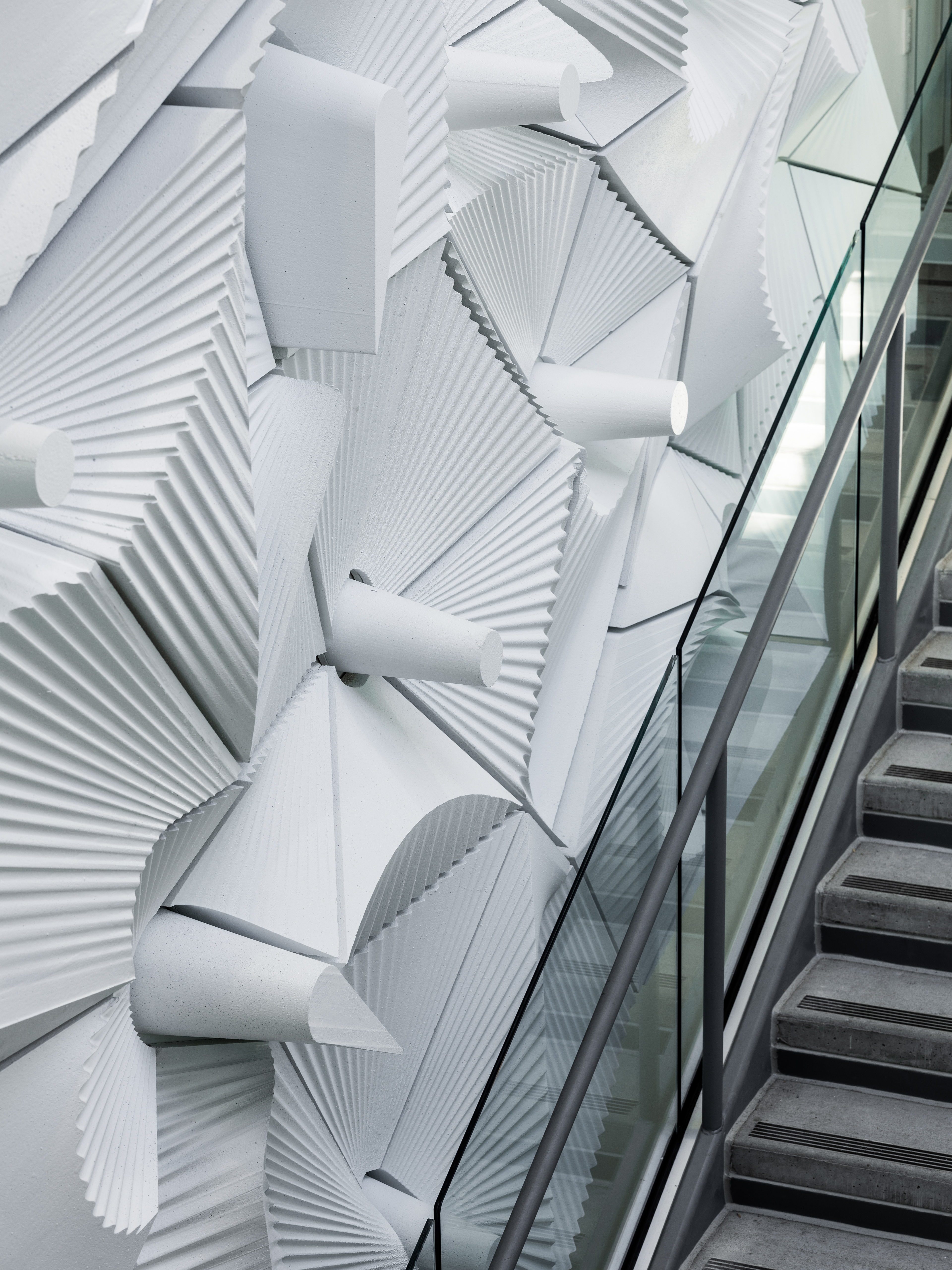
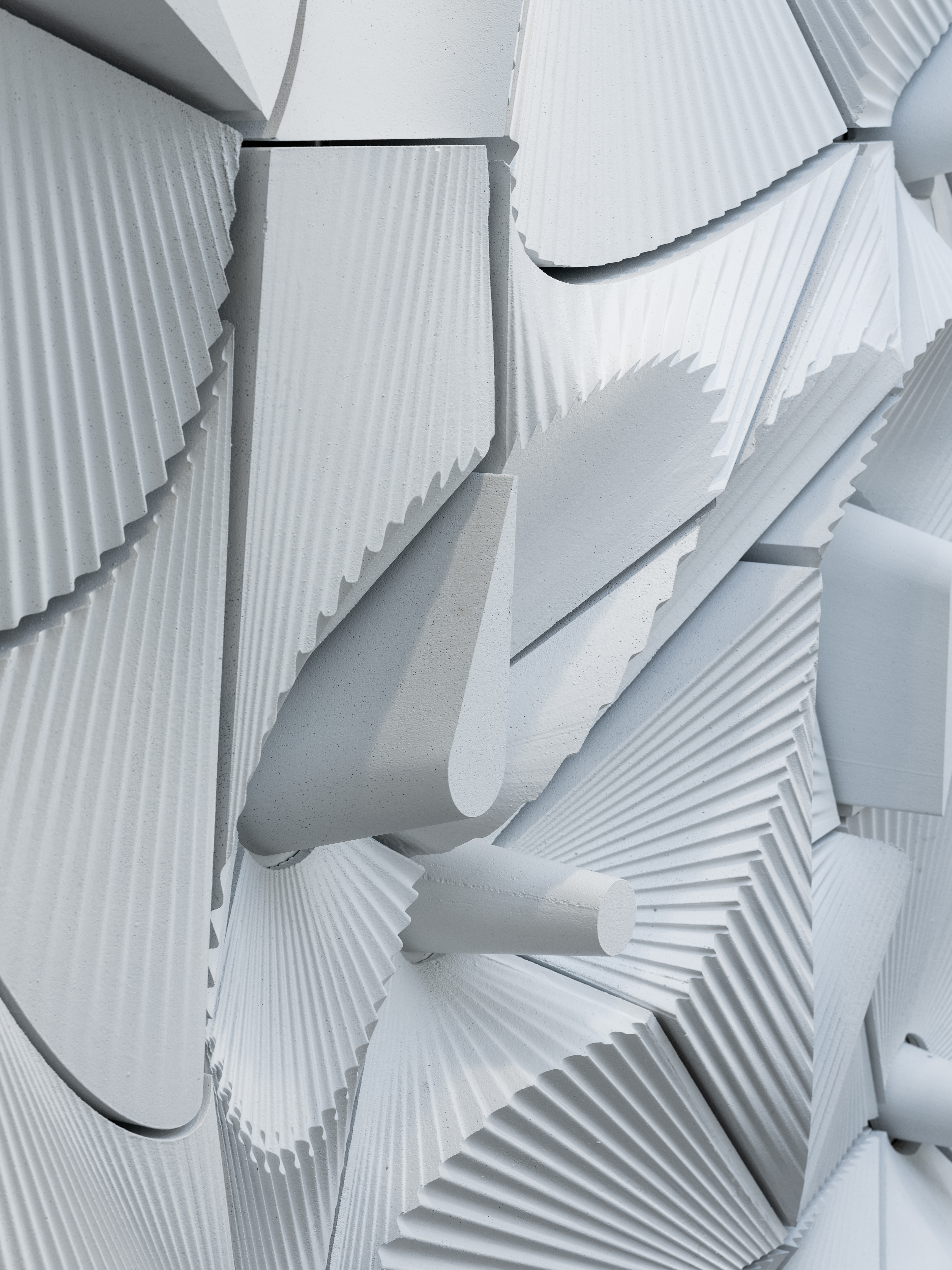
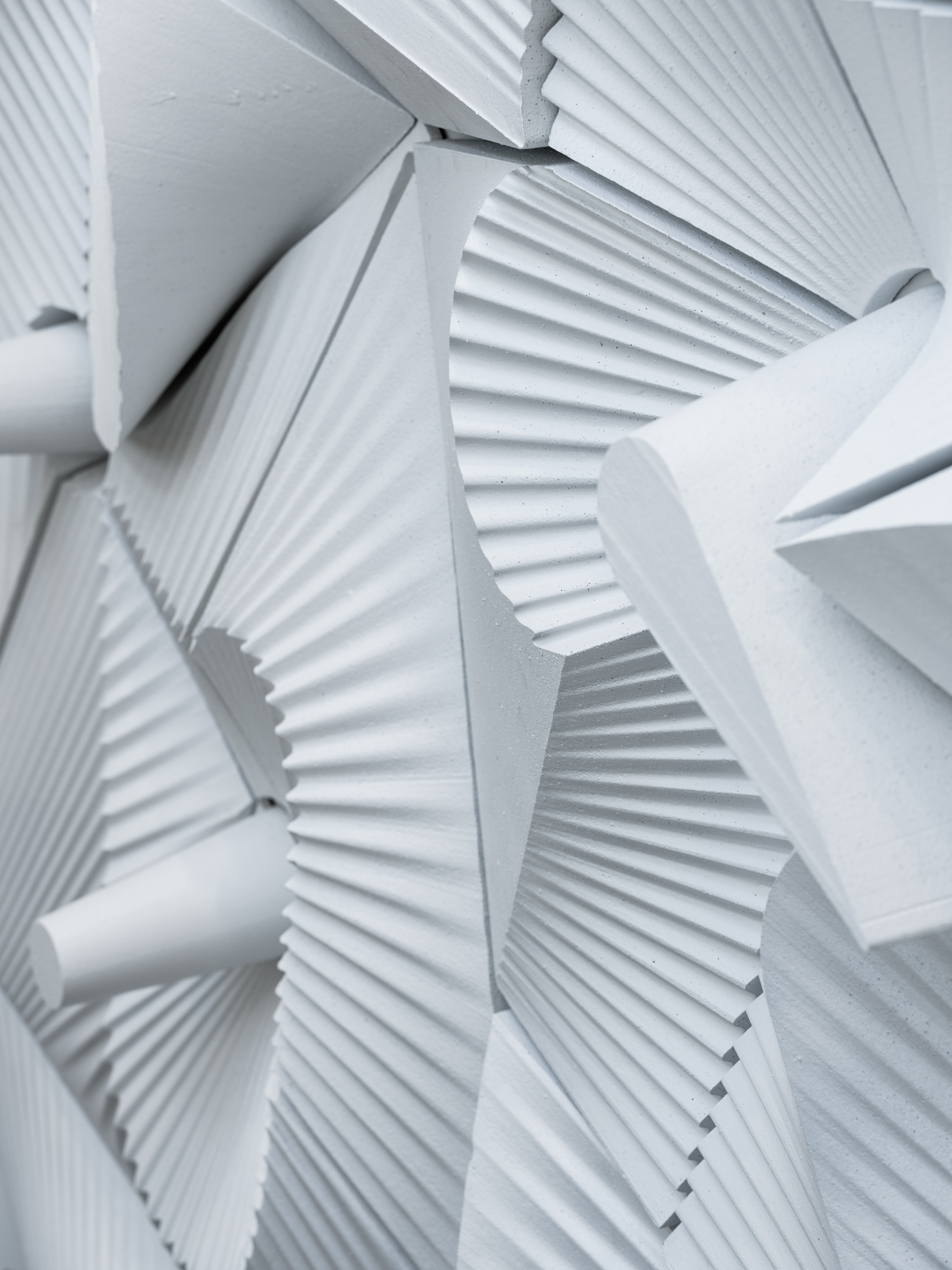
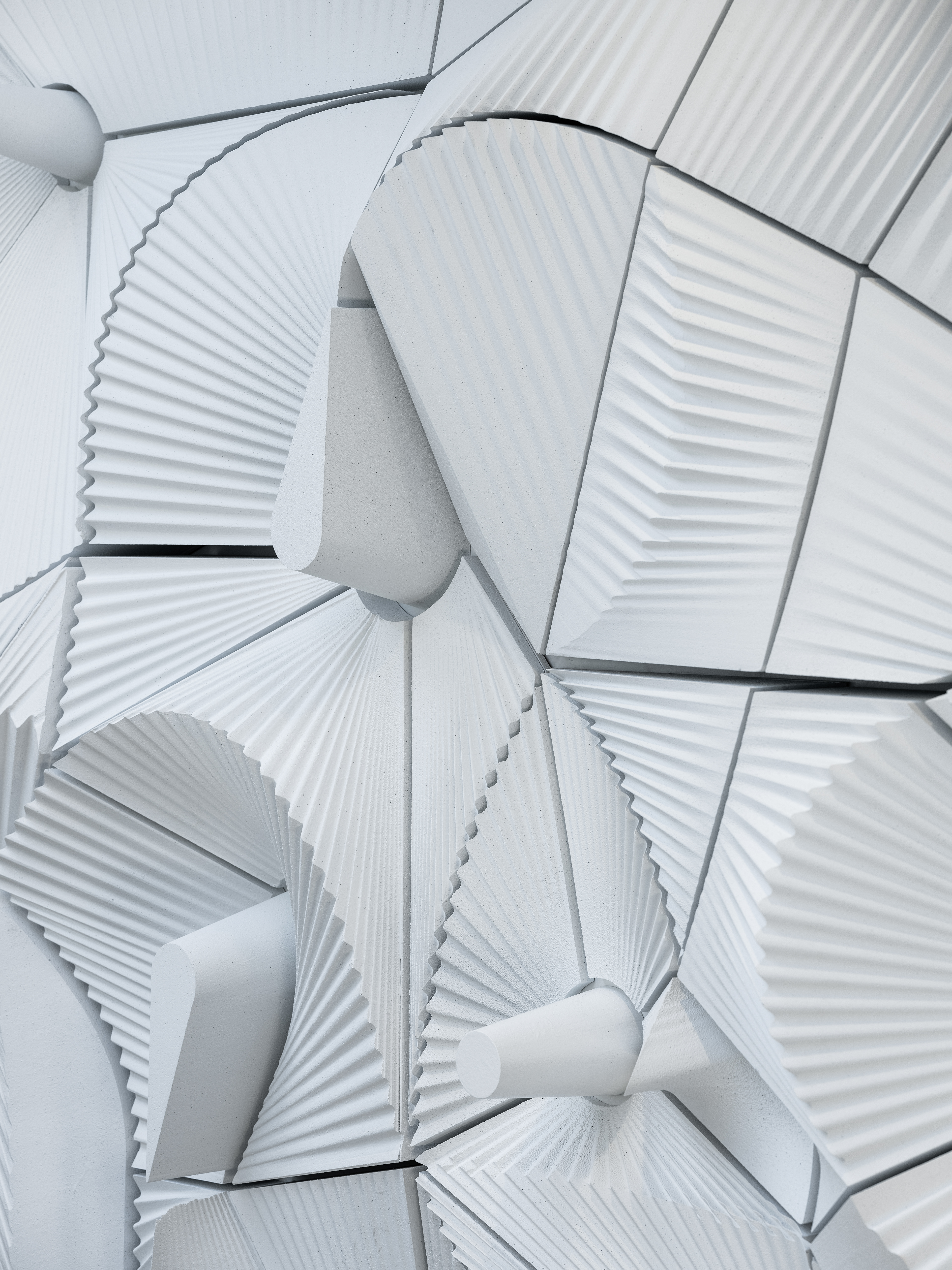
Neural Networks + Explicit ModelinG
Variation 1
Variation 2
Variation 3
Original output from the VGG-19 deep learning neural network and cloud-based computing
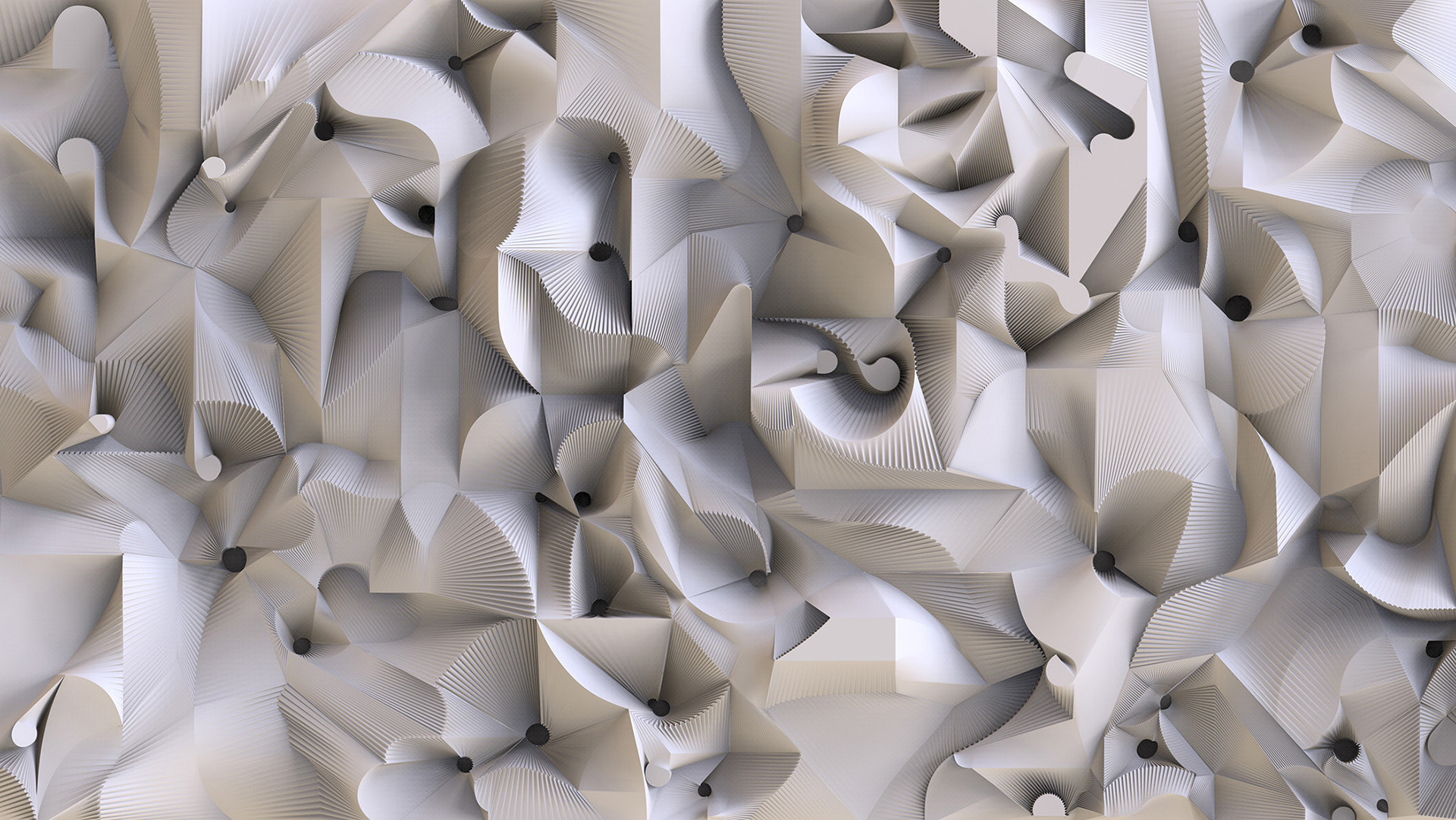
Explicitly modeled interpretation of VGG-19 output
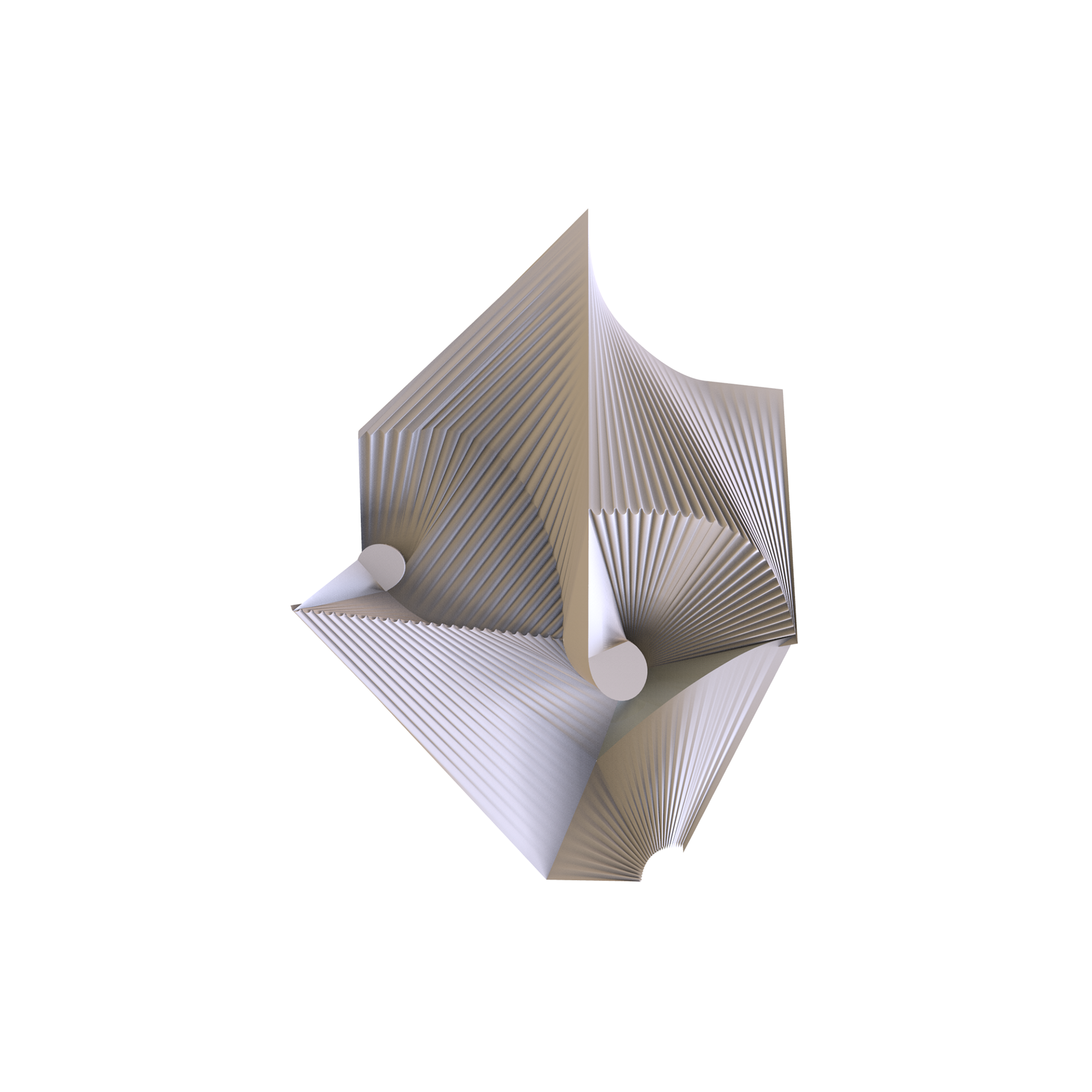
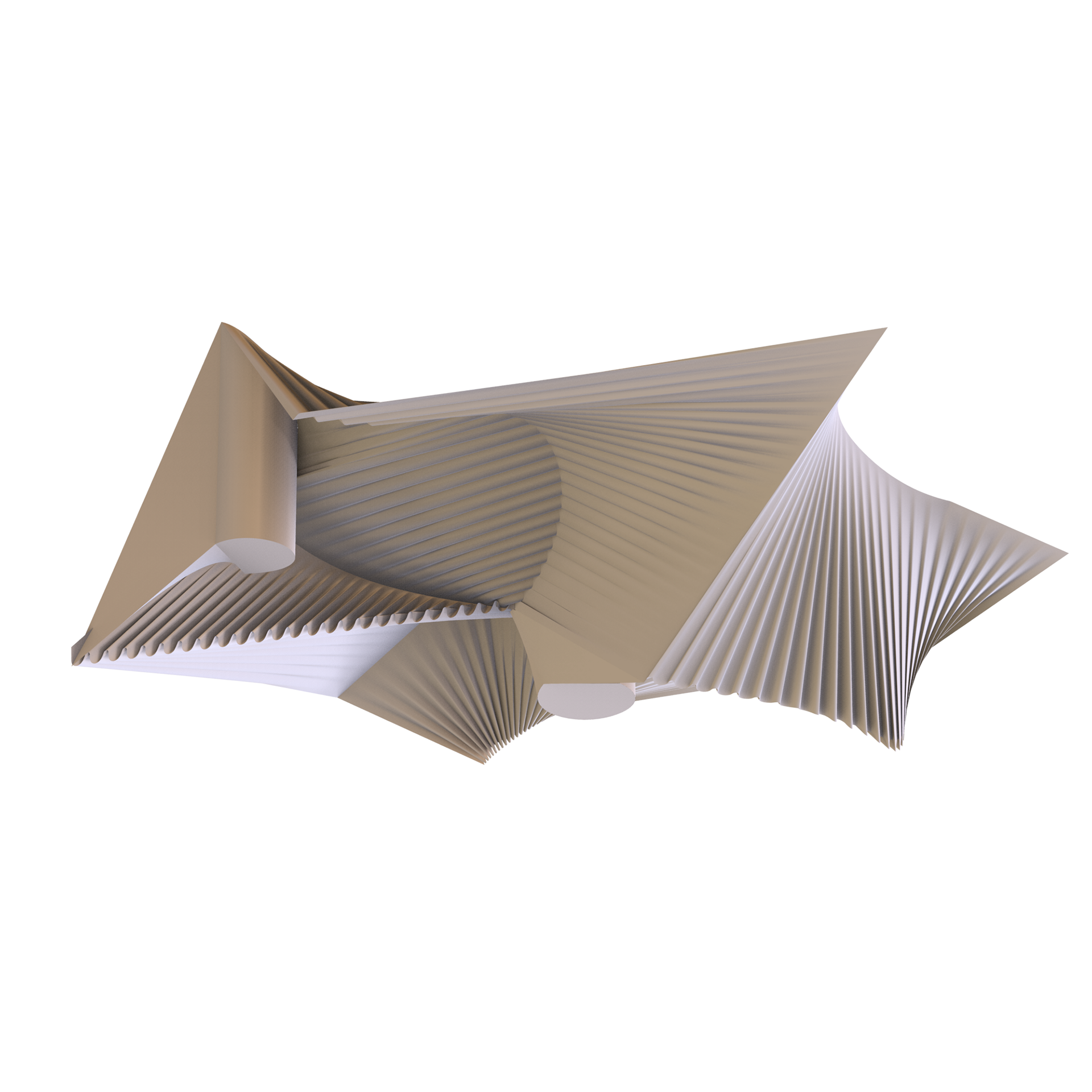
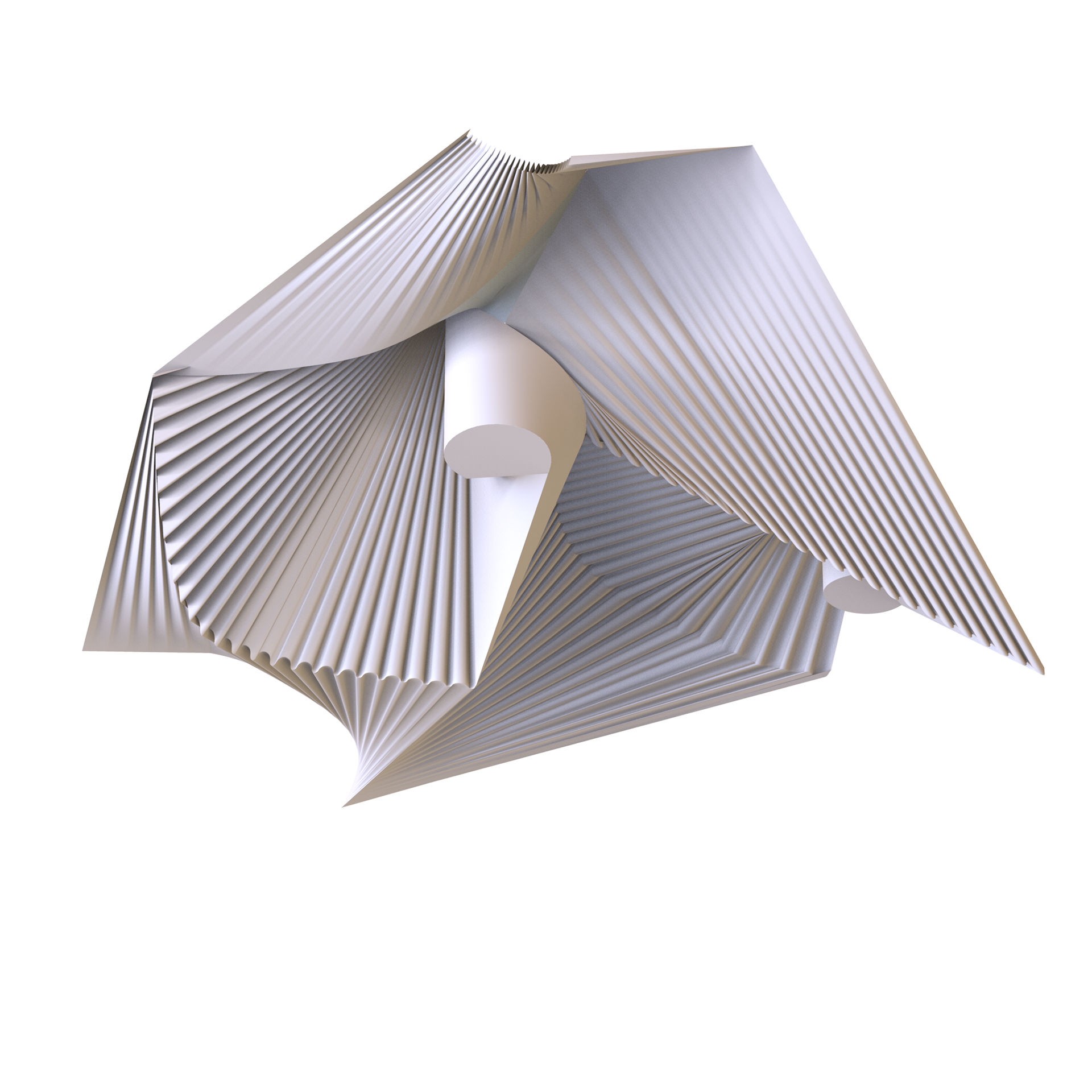
Initial detailed study of explicitly modeled geometry for fabrication
Middletown Relief Proposal
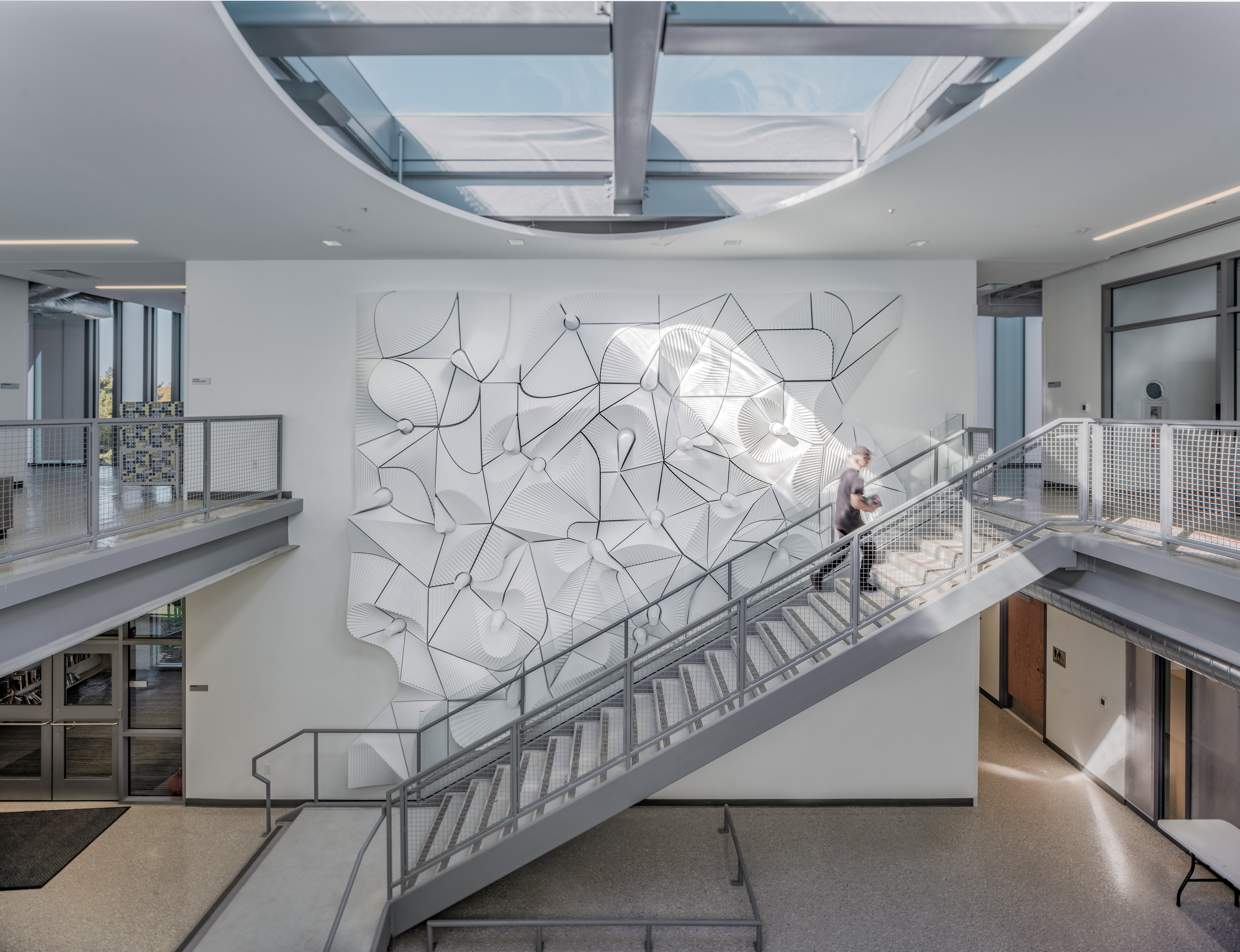
Daylight study of Middletown Relief Proposal
Fabrication process
Example of fabrication process (6-axis robotic hot-wire cutting)
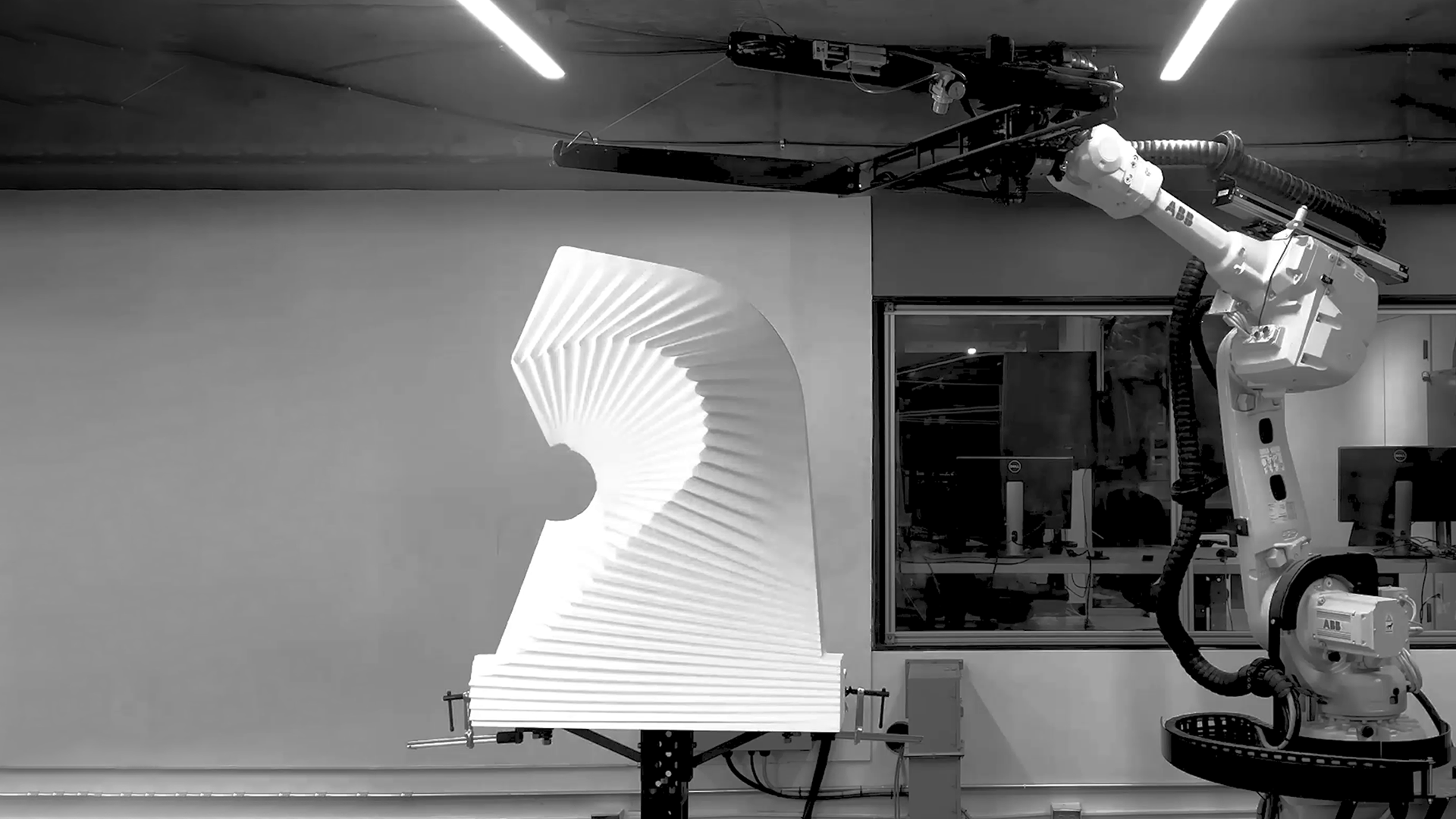
Robotically Control Hot-Wire Cutting
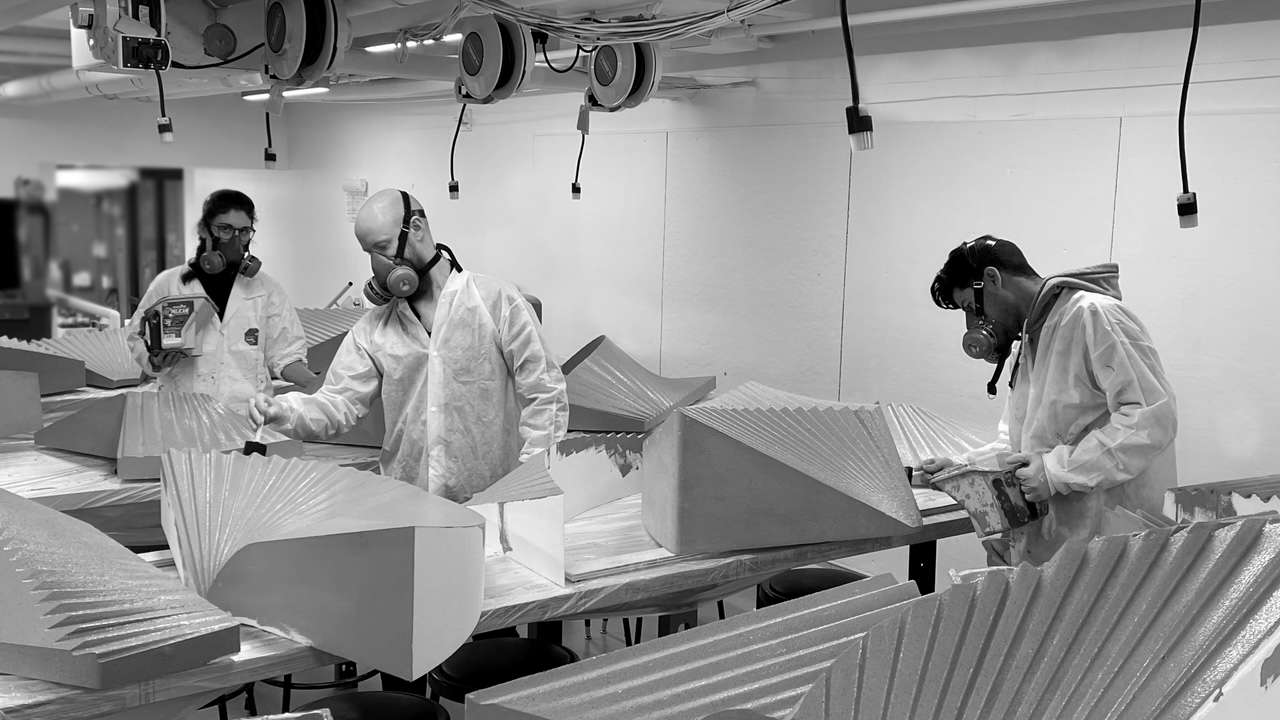
Epoxy Coating of Foam Parts
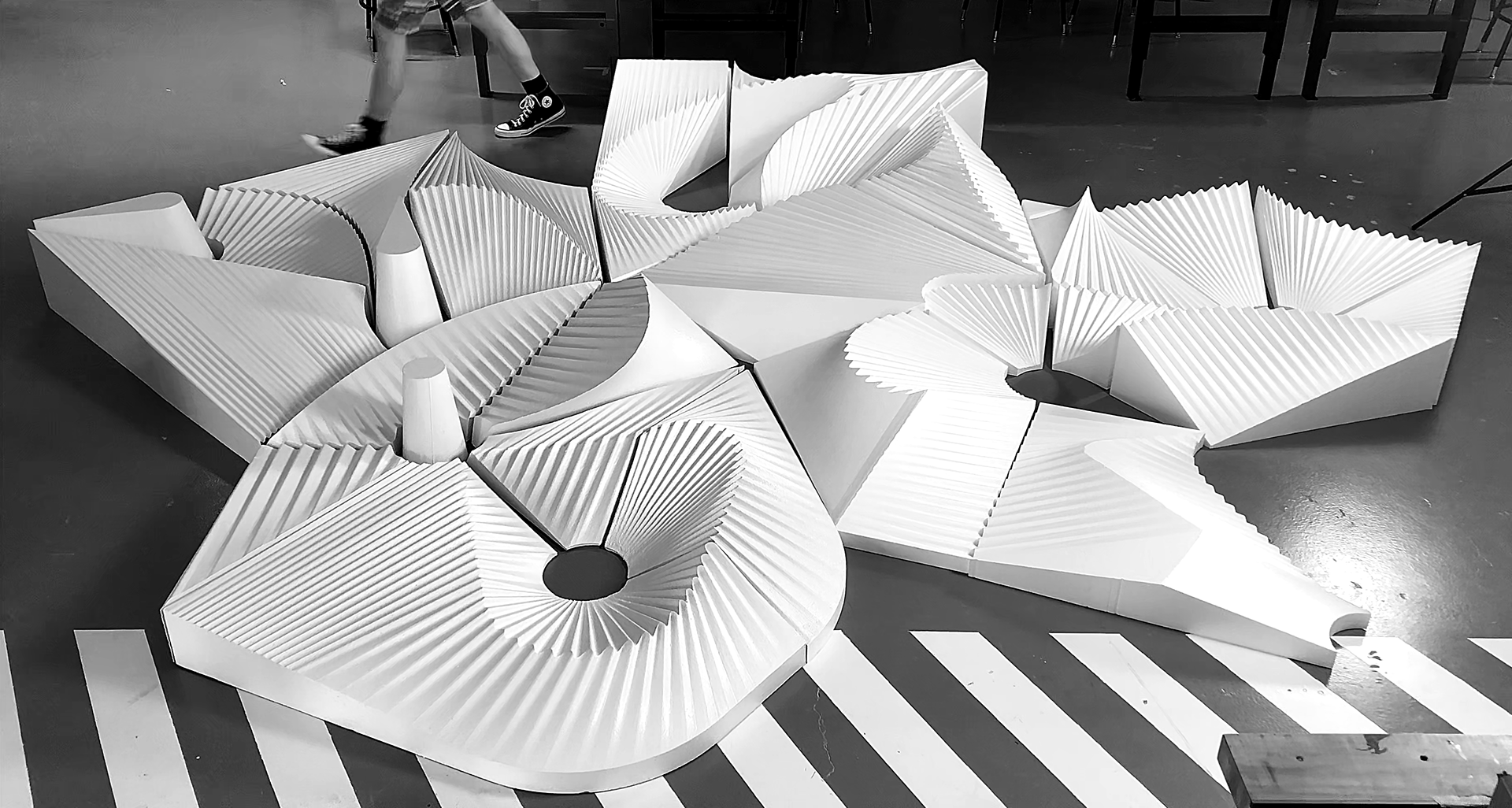
Foam Parts
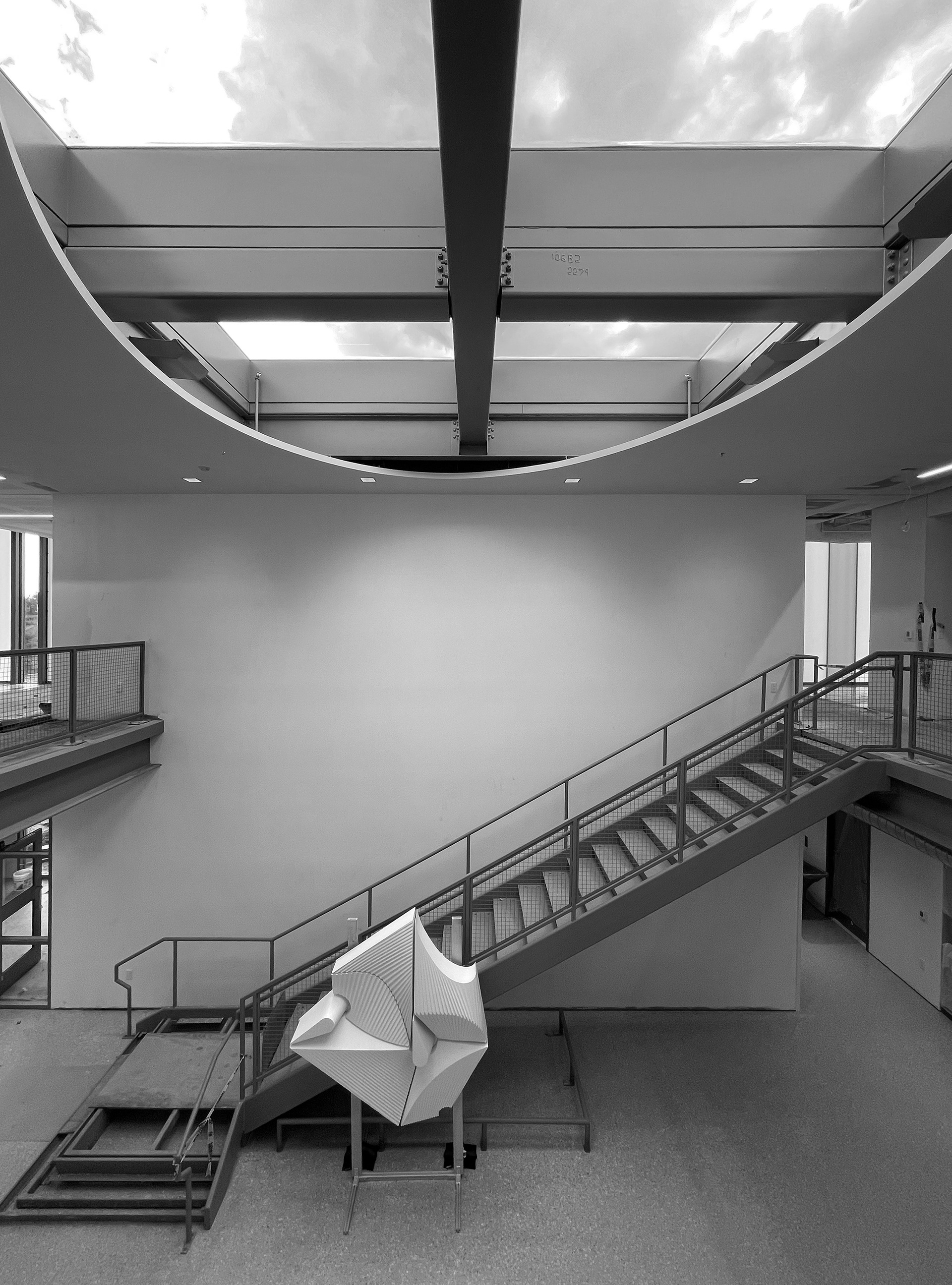
Mockup (In Situ)
Weitzman School of Design
University of Pennsylvania
Penn Praxis Design Fellowship
Faculty: Andrew Saunders
Design Fellows: Matthew White, Riley Studebaker, Caleb Ehly
Jesse Allen, Claire Moriarty, Cecily Nishimura